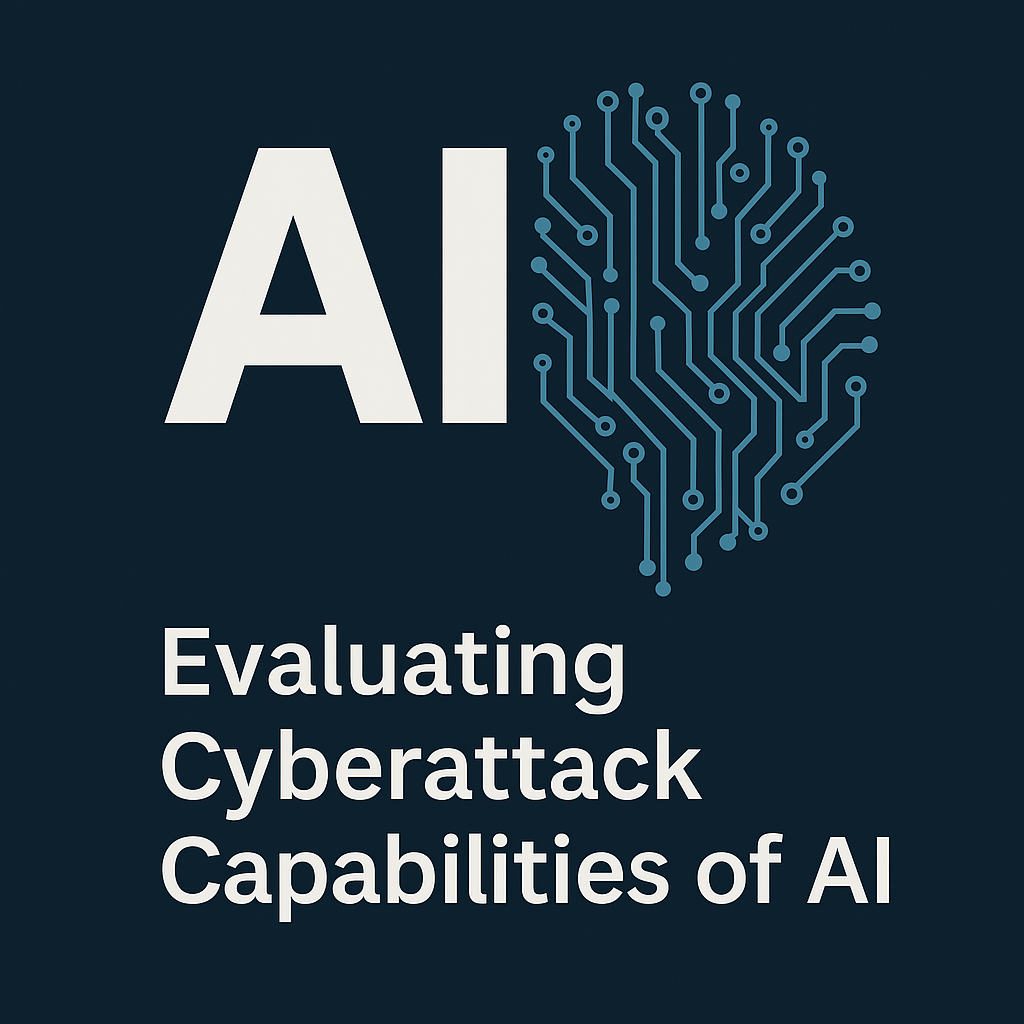
The rise of artificial intelligence (AI) has brought transformative potential across various domains, including cybersecurity. While AI can bolster defenses, it also poses significant risks as an enabler of sophisticated cyberattacks. At the frontier of AI development, understanding these dual capabilities is essential for ensuring safe artificial general intelligence (AGI) development. This article proposes a novel evaluation framework to systematically analyze AI’s role in cyber threats, identifying potential gaps and guiding effective defense strategies. By examining over 12,000 real-world AI instances in cyberattacks, this framework offers valuable insights for prioritizing targeted mitigations and conducting AI-enabled adversary emulation for enhanced security measures.
The Dual Nature of AI in Cybersecurity
AI technologies present both significant opportunities and challenges for cybersecurity. On one hand, AI systems can strengthen defensive capabilities by detecting anomalies, automating threat responses, and identifying vulnerabilities before attackers exploit them. On the other hand, these same technologies can be weaponized to create more sophisticated, efficient, and scalable attack vectors that traditional security measures struggle to counter.
As AI capabilities advance, cybersecurity frameworks must evolve to address new vulnerabilities and attack vectors. This includes expanding established frameworks like MITRE ATT&CK to cover AI-specific techniques and developing new models that account for the unique capabilities of autonomous systems. The future of cybersecurity depends on these frameworks’ ability to adapt to the shifting dynamics between offensive and defensive AI applications.
Understanding and Improving Cybersecurity Frameworks
Despite their effectiveness, traditional frameworks need continuous adaptation to address evolving AI-driven threats. Organizations should implement incremental improvements by customizing frameworks to their specific technology ecosystem and threat landscape. For example, a healthcare provider might prioritize MITRE ATT&CK techniques commonly used against medical records systems, while a financial institution might focus on techniques targeting banking infrastructure.
Cross-functional collaboration enhances framework implementation significantly. Including stakeholders from various departments ensures more comprehensive coverage and practical defense strategies. Organizations should also integrate threat intelligence feeds to keep frameworks current, analyzing emerging attack patterns from multiple sources to identify new techniques before they become widespread.
Automation and orchestration tools can amplify framework effectiveness, enabling real-time mapping of security events to frameworks and accelerating response. Regular tabletop exercises and red team assessments help validate framework implementations against realistic scenarios. Organizations should also develop custom metrics aligned with their risk profiles to measure framework effectiveness.
The Four-Stage Evaluation Framework
The Four-Stage Evaluation Framework bridges the critical gap between identifying AI’s cyber capabilities and translating those insights into practical defense strategies. In Stage One, we begin by curating representative attack chains, analyzing over 12,000 real-world instances of AI use in cyberattacks. This produces a comprehensive “basket” of attack patterns reflecting current threat methodologies across various sectors and adversary types, grounded in actual attack practices rather than theoretical possibilities.
Stage Two involves conducting bottleneck analysis across these attack chains. By examining historical attack data and interviewing security experts, we identify key phases that traditionally require significant attacker resources or specialized expertise. These bottlenecks represent critical junctures where AI could most dramatically reduce attack costs and democratize advanced techniques previously limited to sophisticated threat actors.
In Stage Three, we develop targeted evaluations specifically designed to measure AI’s impact on these bottlenecks. Unlike general capability tests, these evaluations simulate realistic conditions with appropriate constraints and focus on quantifiable metrics like time reduction, success rates, and scalability improvements that directly correlate to real-world attack economics.
Finally, Stage Four executes these evaluations to generate cost differential scores, revealing how significantly AI might disrupt attack economics across different phases. This approach provides defenders with actionable intelligence on where to prioritize defensive investments against emerging AI-enabled threats.
Translating Findings into Defense Strategies
The fundamental challenge in cybersecurity’s AI era is bridging the gap between capability evaluations and actionable defense strategies. Our framework addresses this through four interconnected mechanisms that transform theoretical risk assessments into practical security measures.
First, threat coverage gap assessment maps AI capabilities to specific attack chain phases, revealing where defenses need reinforcement. Our evaluations found significant AI advantages in operational security functions (40% success rate), particularly in evasion techniques relevant to Installation and Command & Control phases. This helps security teams prioritize defenses against techniques where AI shows particular strength, such as living-off-the-land tactics and encrypted communications.
Second, organizations must develop targeted mitigations based on these identified gaps. Following Google DeepMind’s Frontier Safety Framework approach, this involves implementing tailored safeguards—from model-level controls like safety fine-tuning to operational mitigations like enhanced detection rules. Crucially, these safeguards undergo rigorous assessment through assurance evaluations, threat modeling, and formal safety cases to verify their effectiveness against evolving AI-enabled threats.
Third, the framework significantly enhances adversary emulation by enabling red teams to model AI-augmented attackers more accurately. By synthesizing knowledge about traditional adversary TTPs with evidence of AI-enabled cost reductions across attack phases, teams can create realistic emulation scenarios that test defenses against emerging hybrid threats.
Finally, the framework enables benchmarking defense effectiveness by quantifying how security controls impose costs on AI-enabled attacks. Since effective cyber defense fundamentally aims to increase adversary costs, measuring this impact across the attack chain provides a concrete method for assessing intervention value and justifying security investments in an increasingly complex threat landscape.
Conclusions
This article presents a novel framework for evaluating AI’s cyber capabilities across the entire attack chain, revealing significant insights for defense strategies. Our evaluations show that current AI assessments often overlook critical stages like evasion and persistence where AI demonstrates notable potential. By continually updating our attack chains and bottleneck analyses based on real-world misuse cases, this framework equips defenders to better understand AI-driven attack costs and prioritize mitigations. Ongoing community collaboration is crucial to adapt our defenses against the evolving capabilities of AI, ensuring cybersecurity measures remain robust in the face of new threats.